In Q3 2023, FinregE launched its large language model, the Regulatory Insights Generator (RIG). This model has been in development since 2021 in collaboration with Imperial College, London, as part of FinregE’s SMART project funded by UK Innovate.
The goal of this project was to build a natural language processing-driven model focussed on legislative and regulatory content classification, key information and obligations extraction, and summarized interpretations of laws and rules.
RIG is the first LLM in the market that is specifically trained to act like a regulatory expert and answer questions accurately on regulations for compliance purposes. It is also the first LLM in this space that does not use OpenAI.
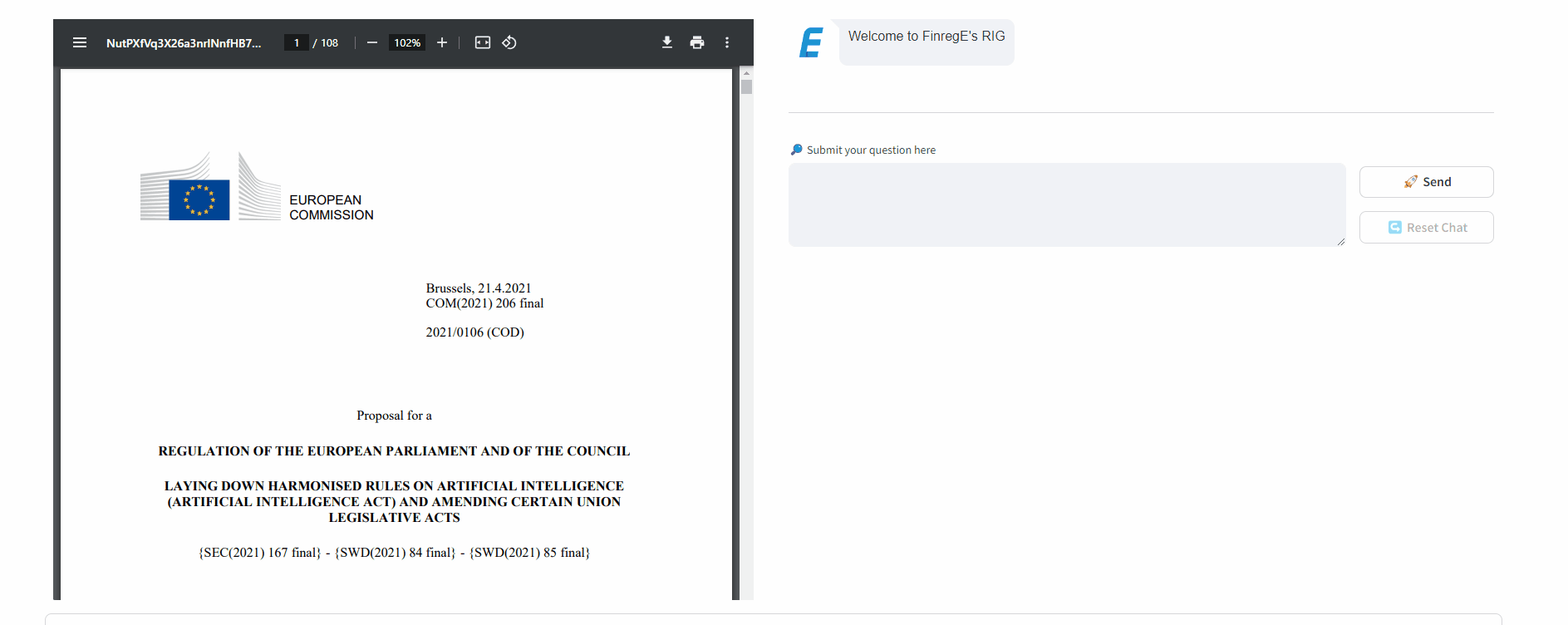
With financial services seeking to leverage AI for regulatory, legal and compliance purposes, we went head-to-head with ChatGPT and Bard to test and provide RIG’s strength in regulatory analysis.
In this blog we cover:
- Why create RIG?
- RIG vs ChatGPT, Bard and others: What’s the difference?
- RIG vs ChatGPT: Key test questions using the EU AI Act
- RIG vs ChatGPT: Key test findings
- RIG vs ChatGPT: Full test results
- Start using RIG
Why create RIG?
FinregE’s regulatory compliance software provides an intelligent and user-friendly platform that serves as a centralized library of global regulatory laws and publications, updated continuously in real-time.
Financial institutions, regulators, and other stakeholders often struggle with fragmented and outdated regulatory content from myriad sources. Teams waste countless hours digging through lengthy documents across disparate systems to identify relevant rules. By the time they extract key obligations and requirements, some of the extracted information may already be obsolete due to amendments.
The FinregE platform alleviates these challenges by consolidating regulatory content into a single location, ensures it remains current. The platform monitors official sources globally for any modifications made to existing regulations. It then programmatically updates relevant records, allowing users to trust they are referencing the latest guidelines.
Additionally, FinregE leverages natural language processing capabilities to analyze incoming regulatory changes and identify critical new requirements. This protects users from overlooking crucial obligations among pages of modifications. The platform also categorizes requirements, facilitates search, and enables comparisons across regulations and jurisdictions.
FinregE clients asked us to expand our platform’s AI and NLP capabilities to:
- Summarize regulations to highlight key purpose and intended outcomes
- Extract and connect regulatory requirements across single or multiple regulations to clarify required actions
- Analyze new and updated regulations and build project plans for efficient implementation
- Cross check clients’ existing control frameworks to identify gaps compared to latest regulatory obligations
- Recommend optimized control frameworks mapped to requirements
- Link regulatory texts to compliance policies, procedures, and controls for greater transparency
RIG vs ChatGPT, Bard and others: What’s the difference?
As we launched RIG and provided access to clients, one frequent question was “Why use RIG over GPT?”
The key difference between RIG and ChatGPT/other LLMs is the data used to train the RIG LLM. RIG is trained on focused legal and regulatory training set from FinregE regulatory library of global regulatory laws and publications. In addition, RIG’s training continually kept up to date whereas ChatGPT’s data hasn’t been updated since 2022.
RIG was designed with FinregE’s client needs at its centre : specialized regulatory expertise, explainable insights, and ethical AI safeguards.
FinregE | ChatGPT | Bard | Claude | |
Data Freshness | Real time | 2022 | Real Time | 2023 |
Purpose | Specialized regulatory advisor, leveraging extensive legal and compliance training to provide institutions with tailored insights | Conversational agent, designed to interact with users in a natural, human-like manner. | AI chatbot for assisting with information, communication and creativity | Natural conversations and be of use for a wide variety of tasks like answering questions, summarizing text, doing math, and more. |
Training Set | Curated historical data of laws, regulations and regulatory publications from over 150 countries | Websites, books, articles, forums and other information available on the internet | Publicly available datasets, Google’s internal data and data from third-party sources | Not disclosed |
Data Privacy | Data isolation within client instance. No external data transfer or sharing | Includes measures for data privacy with policies in place regarding data handling | Google collects Bard conversations and uses the data to provide, improve, and develop Google products | Use materials to provide, maintain, and improve the Services and to develop other products and services |
Explainability | Answers retrieved from domain specific regulations source material with minimised hallucinations | No explainability of source data of answers | No explainability of source data of answers | Can provide references when prompted of where data is sourced from |
Availability | Live (Gamma testing – under client testing) | Live | Beta | Beta |
RIG versus Other LLMs : Other Key Differences
- Specialized Legal and Regulatory Training: Unlike generic models, RIG was explicitly trained on FinregE’s extensive financial regulatory dataset spanning decades of laws, regulations, and official guidance. This breadth and depth of relevant supervisory content allows RIG to deeply understand regulatory terminology, obligations, and changes over time.
- Tailored Regulatory Compliance Insights: Rather than wide-ranging text generation focused on human-like responses, RIG was optimized to serve as an advisor for regulatory professionals. The model identifies compliance requirements, draws connections to controls, evaluates adherence, and determines where procedures need updating given regulatory amendments.
- Confined Analysis and Privacy: By constraining analysis strictly to texts authorized users provide; RIG inherently respects confidentiality boundaries set by firms. RIG will not incorporate or disclose information beyond the permissions explicitly granted by querying compliance officers and legal partners.
- Mitigated Bias and Irrelevance: As RIG only considers documents that users submit for a given query, responses avoid introducing unrelated biases that can emerge from general web data. Analyses also prevent illogical extrapolations beyond regulatory texts.
- Immunity to Data Leakage Risks: With RIG referencing the specific documents authorized by the querying organization rather than an interconnected global dataset, using the tool poses no risk of data leakage across client firms. No sensitive information gets shared via the collective training process.
- Explainability and Accountability: Auditors, senior managers, and regulators require explainability to adopt new AI tools in governance workflows. By tracing RIG’s analyses back to specific supplied texts, teams get accountability around recommendations and insights even as output quality continues improving through ongoing specialization.
RIG vs ChatGPT: Key test questions using the EU AI Act
To demonstrate RIG’s capabilities versus GPT, we tested the platforms using the EU AI Act Regulation. We asked questions regulatory and compliance professionals would typically analyse manually when interpreting regulatory impacts including:
- A summary of the regulation.
- A summary of the key regulatory requirements from this document
- Analysis and interpretation of requirements on data and data governance rules within the Act.
- Recommendation of a control framework based on these requirements.
Question 1: Tell me what this document is about?
We asked both RIG and GPT to give a summary of the regulation. You can find the detailed answers at the bottom of this blog.
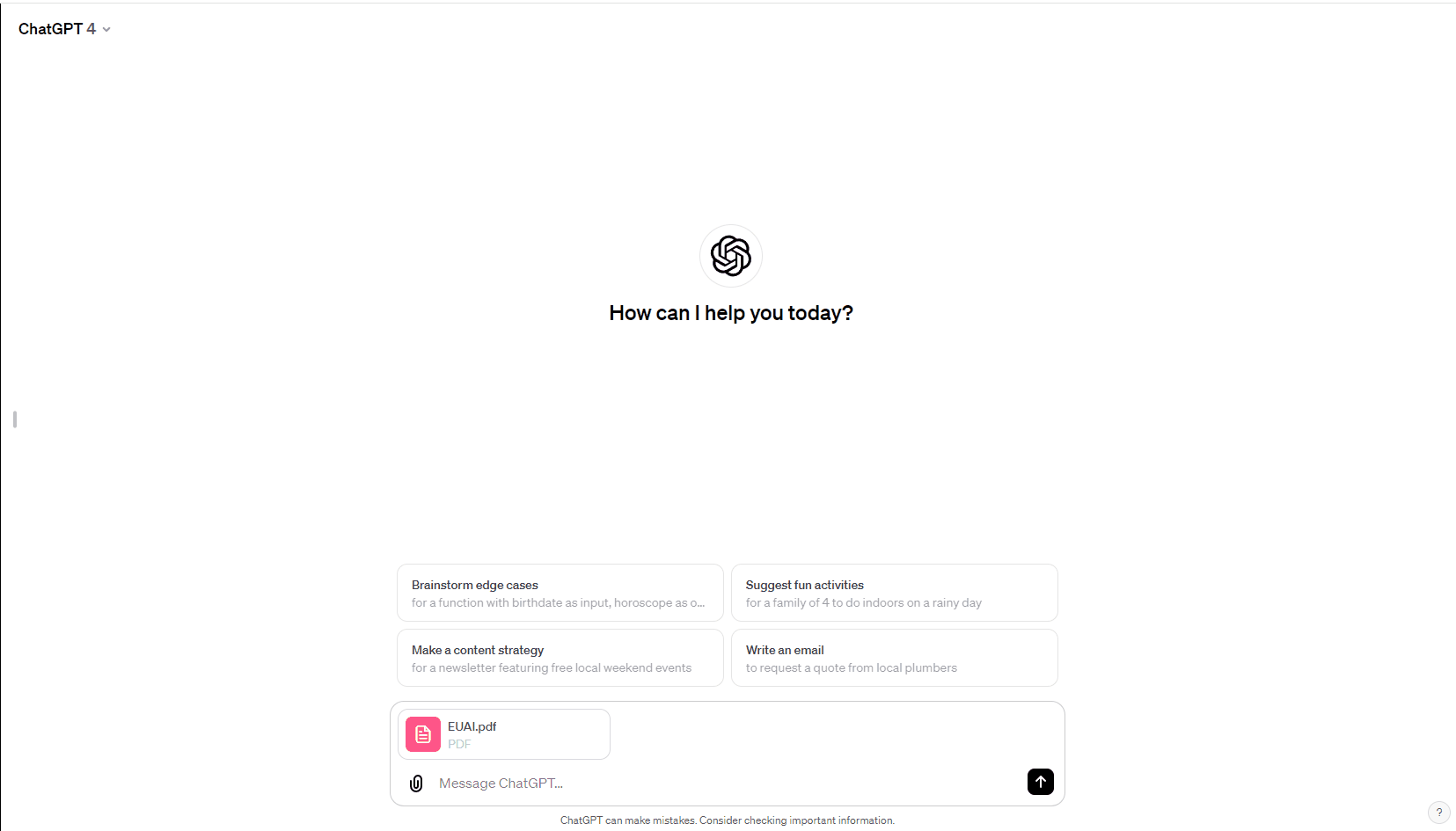
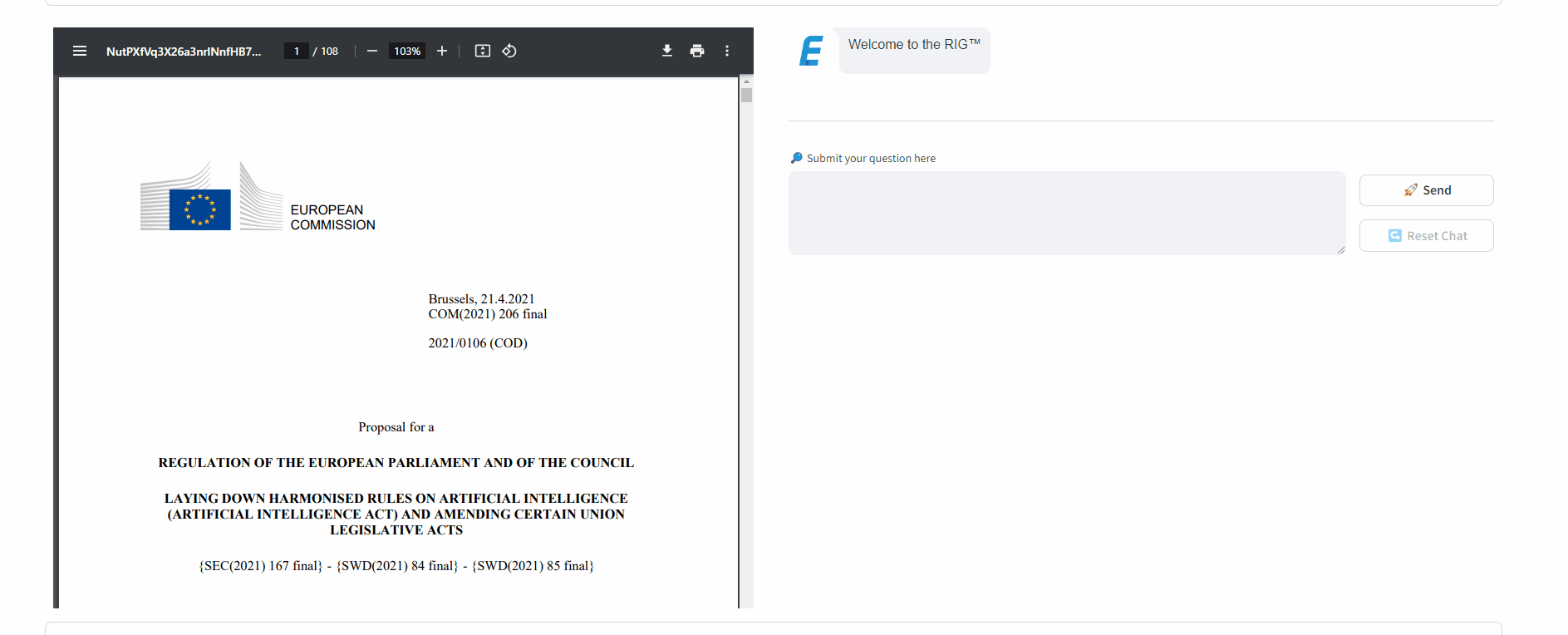
Question 2: Give me a summary of the key regulatory requirements from this document?
In the follow up question, we asked both platforms to give us a summary of key regulatory requirements
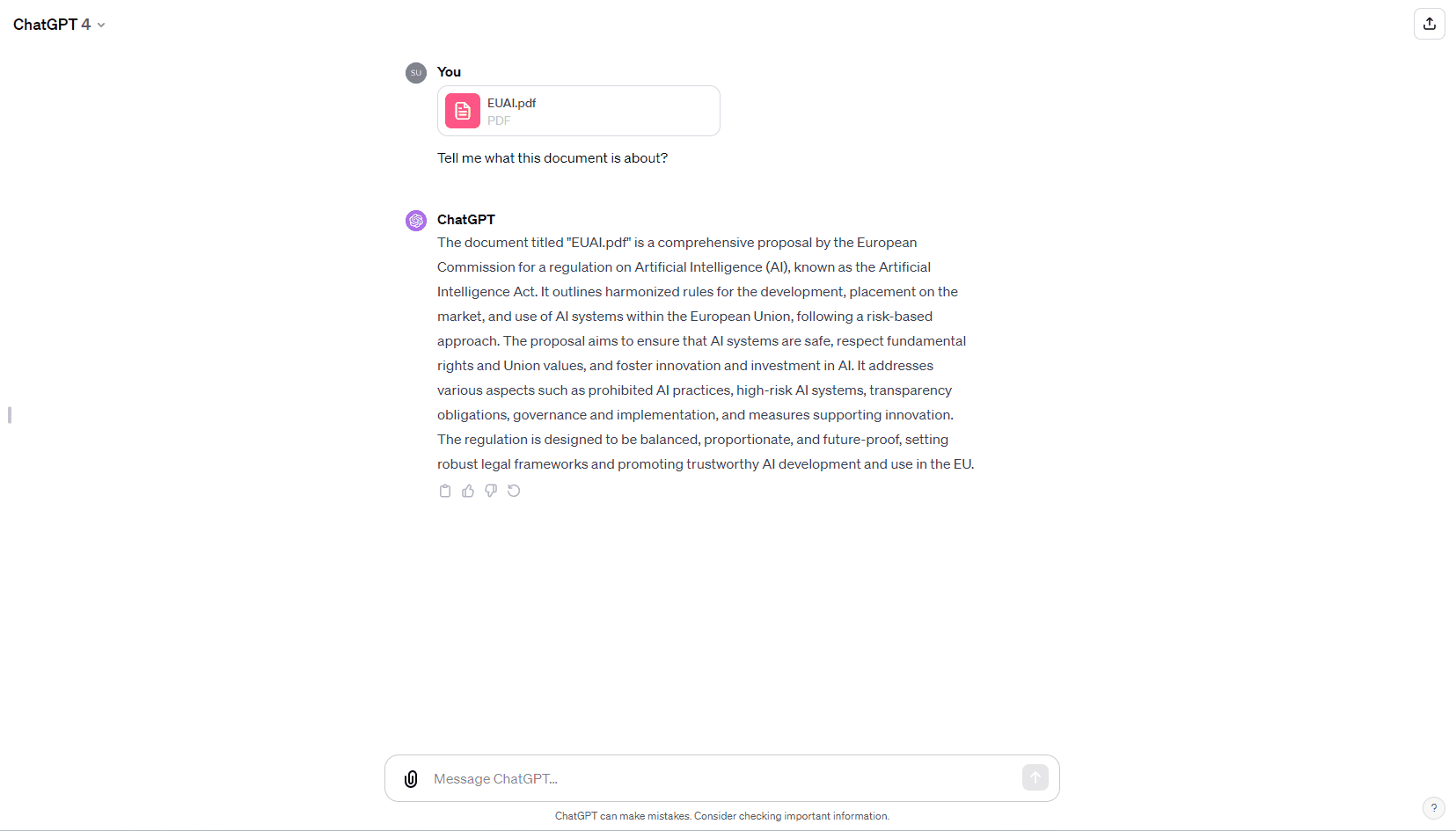
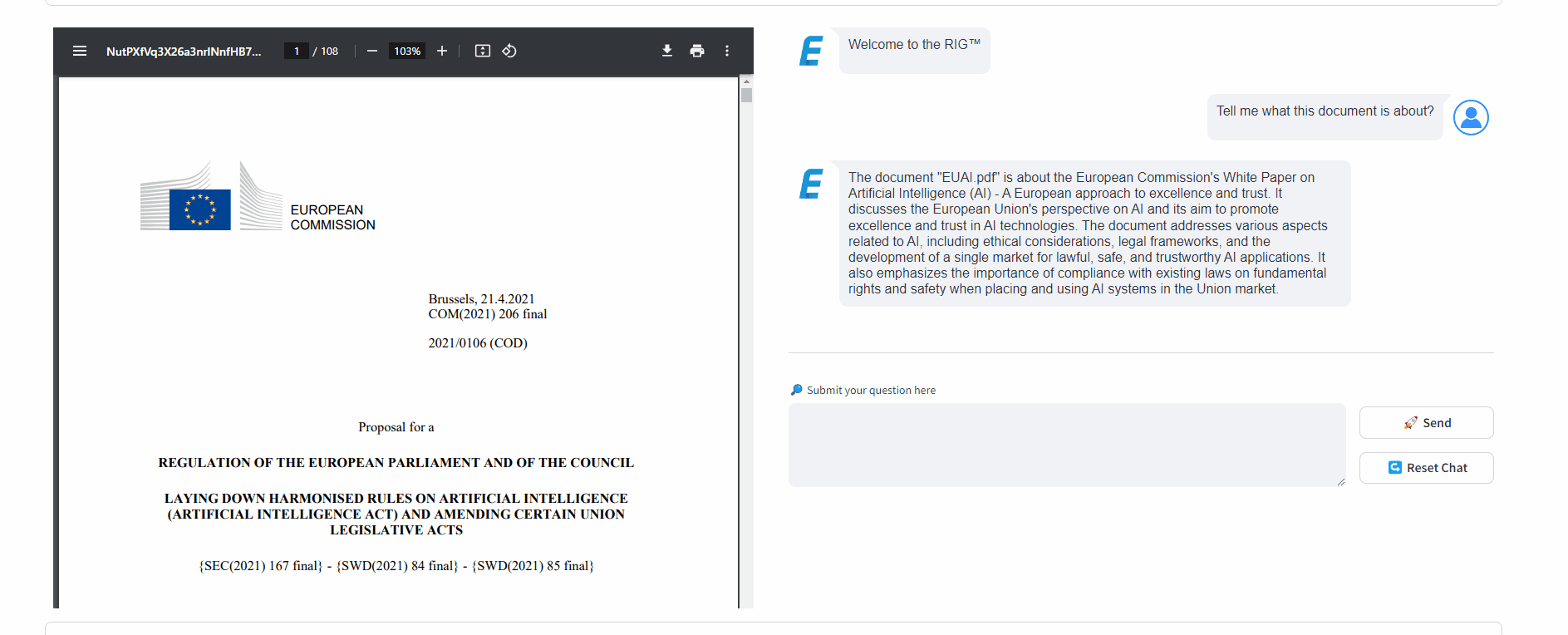
Question 3: What are the requirements on Data and Data Governance?
In the next prompt, we asked both the platforms regarding Data and Data Governance requirements.
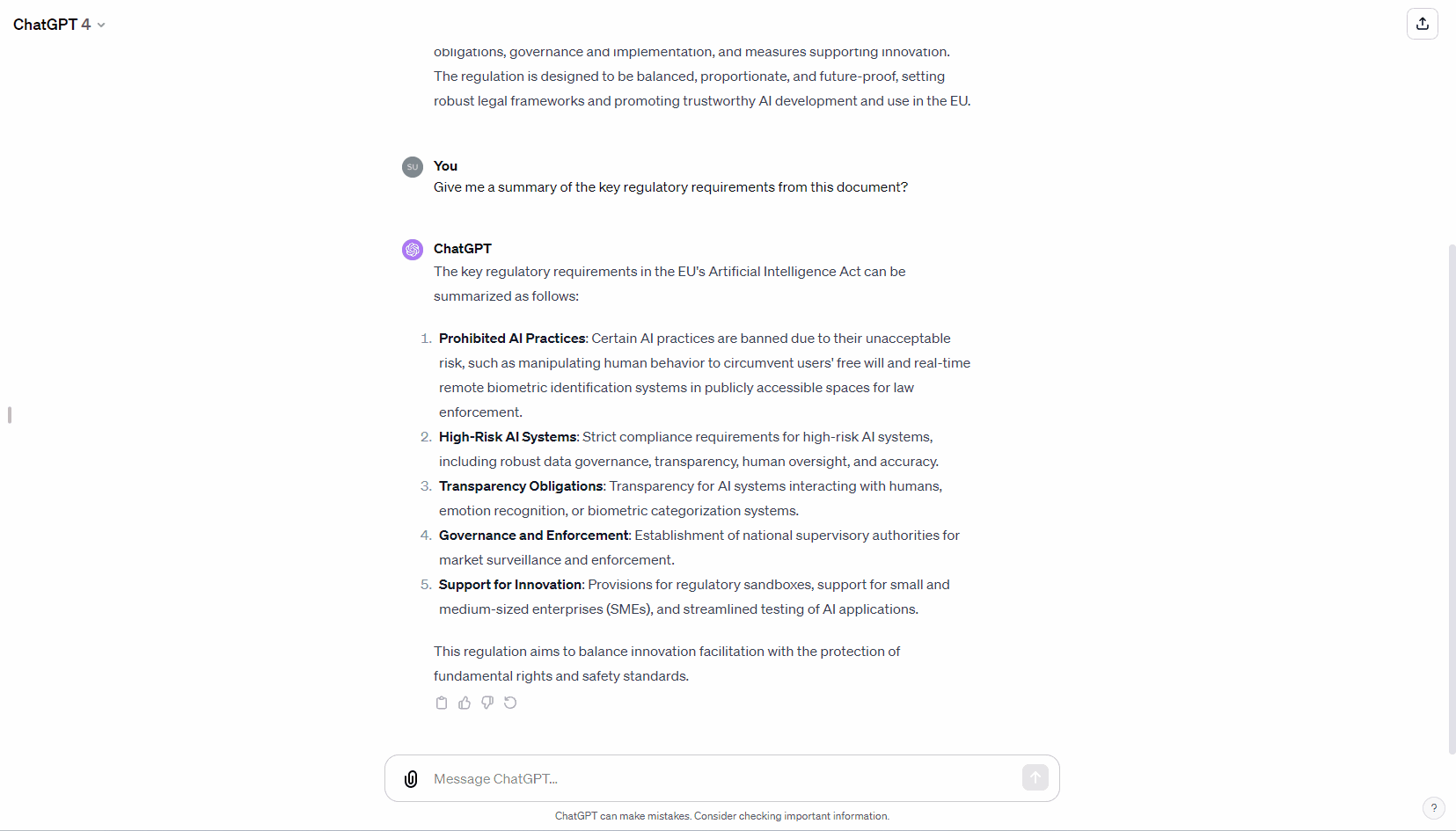
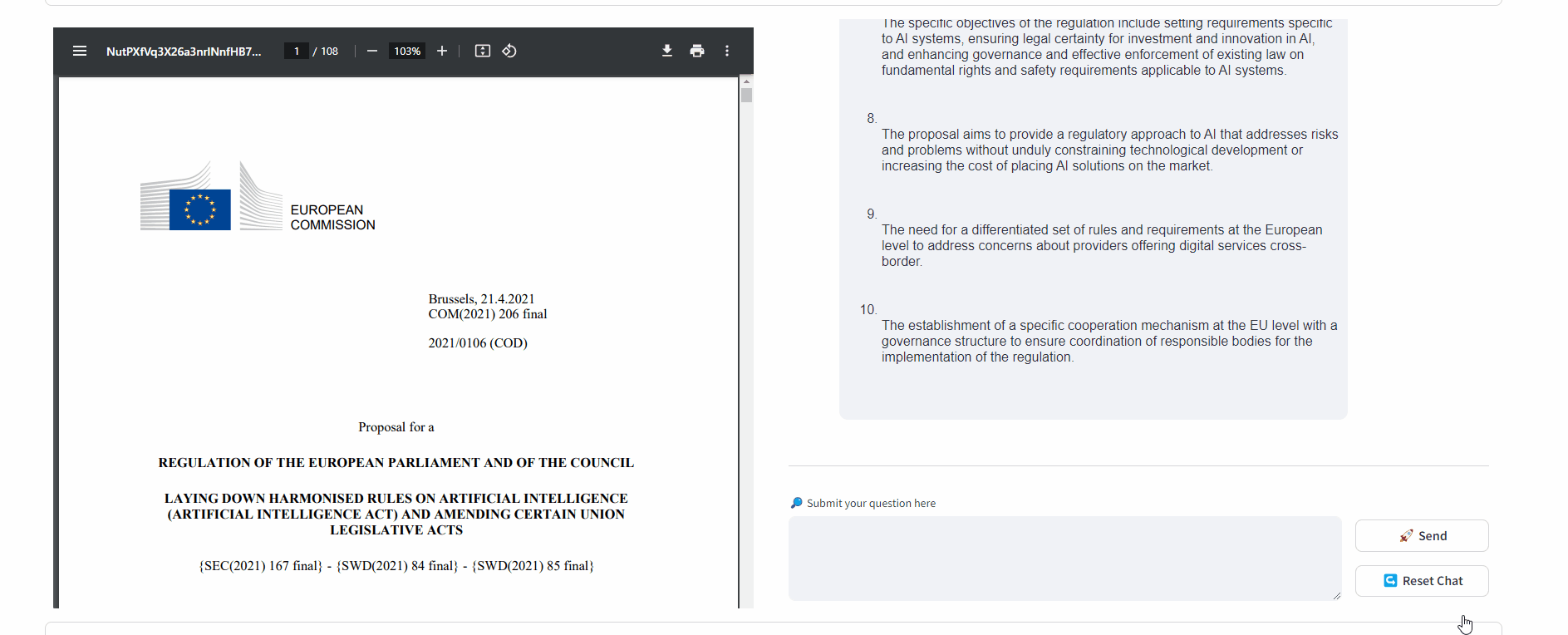
Question 4: Can you recommend a control framework based on these requirements?
In the last prompt, both the platforms were asked to recommend a control framework for the Data and Data governance requirements.
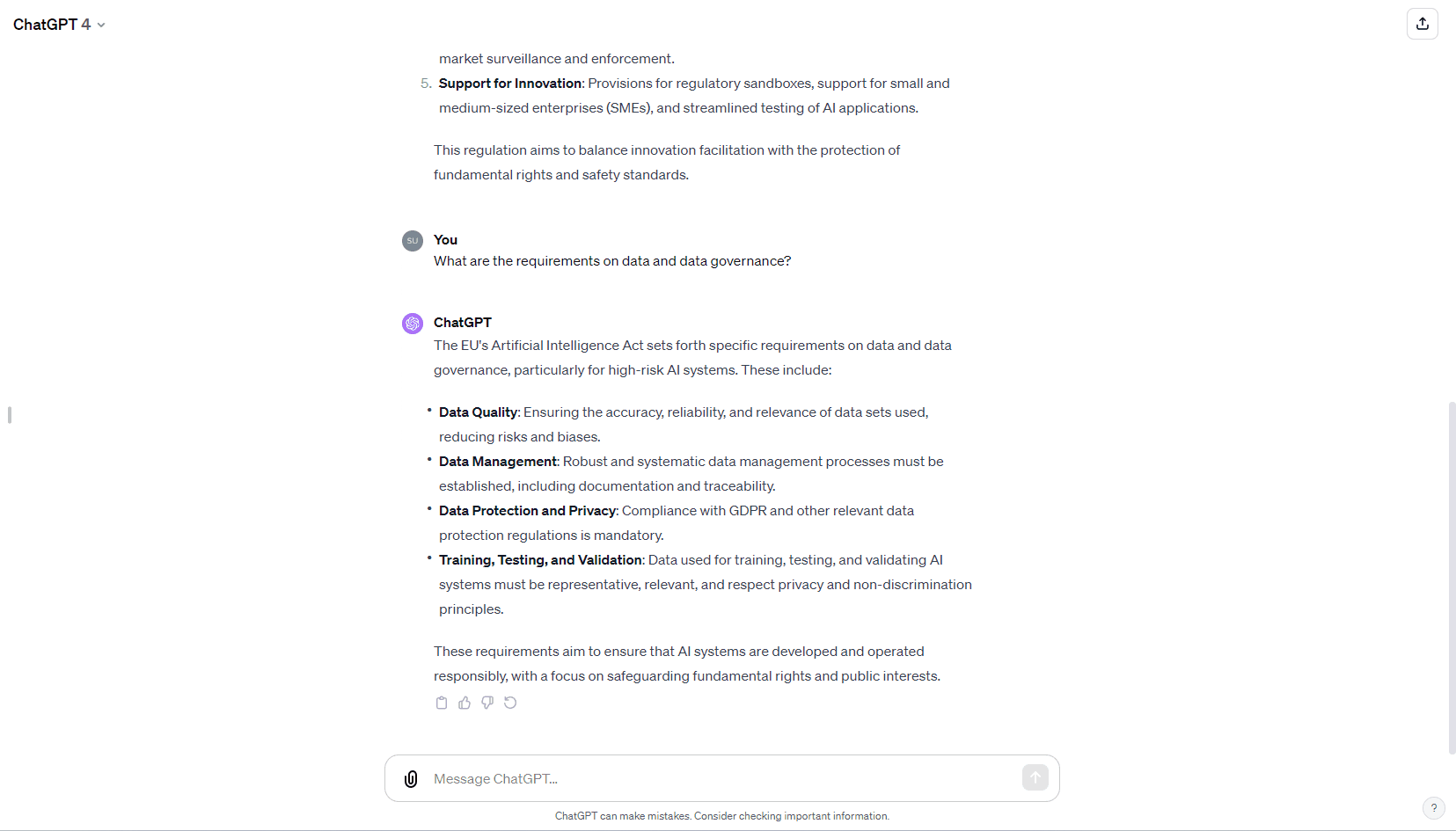
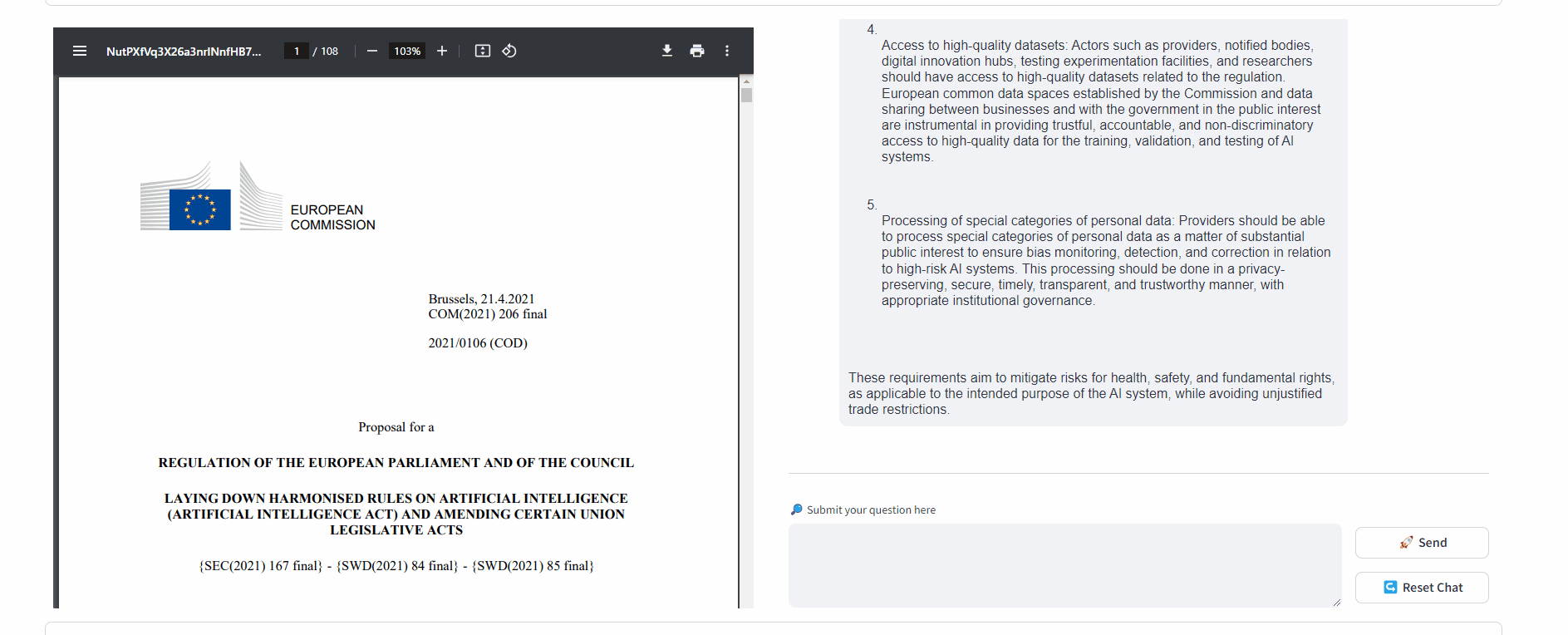
RIG vs ChatGPT: Key test findings
Here are the key takeaways from the test of analysing the results of RIG versus ChatGPT.
- RIG provided comprehensive, actionable regulatory guidance while ChatGPT offered generalized summaries lacking specifics.
- RIG identified precise lists of obligations and changes while ChatGPT responded with eloquent but vague overviews.
- RIG emulated the analytical output compliance professionals require to execute whereas ChatGPT answered conversationally without considering operationalization.
- RIG analysed and presented its results in a methodological manner whereas ChatGPT summerised parts of the regulation using its own analysis.
RIG vs ChatGPT: Full test results
Prompt | RIG Response | GPT Response |
Tell me what this document is about | The document “EUAI.pdf” is about the proposal for a Regulation of the European Parliament and of the Council laying down harmonized rules on artificial intelligence (Artificial Intelligence Act). The purpose of this proposal is to establish a legal framework for trustworthy AI that is based on EU values and fundamental rights. The objective is to promote the uptake of AI while addressing the risks associated with certain uses of the technology. The proposal aims to give people and other users confidence in embracing AI-based solutions, while encouraging businesses to develop them. The rules for AI in the Union market or affecting people in the Union should be human-centric, ensuring safety and compliance with the law, including the respect for fundamental rights. | The document “EUAI.pdf” is about the proposal for a Regulation of the European Parliament and of the Council laying down harmonized rules on artificial intelligence (Artificial Intelligence Act). The purpose of this proposal is to establish a legal framework for trustworthy AI that is based on EU values and fundamental rights. The objective is to promote the uptake of AI while addressing the risks associated with certain uses of the technology. The proposal aims to give people and other users confidence in embracing AI-based solutions, while encouraging businesses to develop them. The rules for AI in the Union market or affecting people in the Union should be human-centric, ensuring safety and compliance with the law, including the respect for fundamental rights. |
Give me a summary of the key regulatory requirements from this document? | The key regulatory requirements from this document include: 1. Providers of high-risk AI systems must have a quality management system in place that complies with Article 17. 2. Providers must draw up the technical documentation of the high-risk AI system. 3. Providers must keep the logs automatically generated by their high-risk AI systems when under their control. 4. High-risk AI systems must undergo the relevant conformity assessment procedure before being placed on the market or put into service. 5. Providers must comply with the registration obligations referred to in Article 51. 6. Providers must take necessary corrective actions if the high-risk AI system is not in conformity with the requirements set out in Chapter 2 of the document. 7. Providers must inform the national competent authorities and, where applicable, the notified body of any non-compliance and corrective actions taken. 8. Providers must affix the CE marking to their high-risk AI systems to indicate conformity with the Regulation. 9. Providers may be requested to demonstrate the conformity of the high-risk AI system with the requirements set out in Chapter 2 of the document. 10. Providers must have a quality management system in place that ensures compliance with the Regulation. 11. Providers must put in place a strategy for regulatory compliance, including compliance with conformity requirements. 12. Providers must draw up technical documentation in accordance with Annex IV. 13. Providers must inform national competent authorities and, where applicable, the notified body of any non-compliance and corrective actions taken. 14. Providers must affix the CE marking to their high-risk AI systems to indicate conformity with the Regulation. 15. Providers may be requested to demonstrate the conformity of the high-risk AI system with the requirements set out in Chapter 2 of the document. 16. High-risk AI systems must meet requirements for data quality, technical documentation and record-keeping, transparency and provision of information to users, human oversight, and robustness, accuracy, and cybersecurity. 17. Providers must have an accountability framework setting out the responsibilities of management and other staff with regard to all aspects listed in the document. 18. The implementation of aspects referred to in the document should be proportionate to the size of the provider’s organization. 19. For providers that are credit institutions regulated by Directive 2013/36/EU, the obligation to put a quality management system in place is deemed fulfilled by complying with the rules on internal governance arrangements, processes, and mechanisms pursuant to Article 74 of that Directive. 20. Providers of non-high-risk AI systems are encouraged to create codes of conduct to foster the voluntary application of mandatory requirements applicable to high-risk AI systems. 21. Providers are encouraged to apply additional requirements related to environmental sustainability, accessibility to persons with disabilities, stakeholders’ participation in the design and development of AI systems, and diversity of development teams on a voluntary basis. 22. Standardization and compliance with harmonized standards are important for providers to demonstrate conformity with the requirements of the Regulation. The Commission may adopt common technical specifications in areas where no harmonized standards exist or where they are insufficient. | The “Artificial Intelligence Act” in the EUAI.pdf document outlines several key regulatory requirements, including: 1. Prohibition of Certain AI Practices: Establishes strict rules against specific AI uses that are considered a clear threat to the rights and safety of individuals. 2. Regulations for High-risk AI Systems: Defines criteria for classifying AI systems as high-risk and prescribes compliance requirements for these systems, including data governance, documentation, transparency, human oversight, and robustness. 3. Transparency Obligations for Certain AI Systems: Mandates specific transparency requirements for AI systems interacting with humans or used for emotion recognition, biometrics, or deepfakes. 4. Governance and Enforcement Mechanisms: Sets up governance structures at both the EU and member state levels for oversight, enforcement, and compliance verification. 5. Market Surveillance and Reporting Obligations: Introduces market surveillance mechanisms to monitor the compliance of AI systems and reporting obligations for AI providers and users. These are high-level summaries of some of the key regulatory requirements from the document. For more detailed information, a thorough review of the entire document is recommended. |
What are the requirements on data and data governance? | According to the document EUAI.pdf, the requirements for data and data governance for high-risk AI systems are as follows: 1. High-quality data sets: The data sets used for training, validation, and testing of high-risk AI systems should be of high quality. This means that they should be relevant, representative, free of errors, and complete. The data sets should also have the appropriate statistical properties, taking into account the intended purpose of the system and the specific characteristics of the persons or groups of persons on which the AI system is intended to be used. 2. Data governance and management practices: Appropriate data governance and management practices should be implemented for the development of high-risk AI systems. These practices should ensure that the systems comply with the requirements set out in the regulation. The practices should cover aspects such as design choices, data collection, relevant data preparation processing operations (such as annotation, labeling, cleaning, enrichment, and aggregation), formulation of assumptions, assessment of data availability and suitability, examination of possible biases, and identification and addressing of data gaps or shortcomings. 3. Technical documentation: The technical documentation of a high-risk AI system should be drawn up before the system is placed on the market or put into service. The documentation should demonstrate that the system complies with the requirements set out in the regulation. It should provide national competent authorities and notified bodies with all the necessary information to assess the compliance of the AI system. The technical documentation should include elements specified in Annex IV of the regulation. 4. Record-keeping: Records should be kept to provide information on how high-risk AI systems have been developed and how they perform throughout their lifecycle. This includes documentation on the general characteristics, capabilities, and limitations of the system, algorithms, data, training, testing, and validation processes used, as well as documentation on the relevant risk management system. The technical documentation should be kept up to date. These requirements aim to ensure that high-risk AI systems have high-quality data, are transparent, and can be effectively monitored and assessed for compliance with the regulations. | The document provides specific requirements on data and data governance, particularly for high-risk AI systems. These requirements focus on ensuring the quality of datasets used to develop and train AI systems. Key aspects include: 1. Data Quality: Ensuring the datasets are relevant, representative, free of errors, and complete. This is crucial for the performance of the AI system and to prevent risks associated with poor data quality. 2. Data Management and Documentation: Adequate documentation on data collection, preparation, and processing is required. This includes detailing the methodologies used to ensure data quality and integrity. 3. Bias Detection and Mitigation: There are stipulations for testing, validating, and, if necessary, mitigating any biases in the datasets. This is essential to prevent discriminatory outcomes from the AI system. 4. Data Governance Framework: Establishing a robust data governance framework is mandatory. This includes protocols for data handling, sharing, and access, as well as compliance with relevant data protection laws (e.g., GDPR). 5. Transparency and Traceability: Ensuring transparency and traceability of data sources, the data used, and the data processing methods. This is vital for accountability and for understanding how the AI system makes decisions. 6. Regular Updates: Regular updates and reviews of datasets are required to ensure ongoing relevance and quality, adapting to changes in the environment or target populations. 7. These requirements are designed to promote trustworthiness and reliability in AI systems, particularly those classified as high-risk due to their potential impact on rights and safety. Compliance with these data governance requirements is critical for organizations developing or deploying AI systems in the EU. |
Can you recommend a control framework based on these requirements? | Based on the requirements mentioned in the document, a control framework for regulatory compliance in the context of AI systems can be suggested. This control framework should include the following components: 1. Compliance and Enforcement Mechanisms: Establish a system for ex-ante and ex-post compliance and enforcement mechanisms to ensure that AI systems meet the requirements set by sectorial legislation and the regulations outlined in the document. This can include internal control checks by providers and third-party conformity assessments for certain high-risk AI systems. 2. Competence and Training: Ensure that natural persons assigned with human oversight of AI systems have the necessary competence, training, and authority to carry out their roles effectively. This can be achieved through training programs, certifications, and ongoing professional development. 3. Accuracy and Robustness: Implement measures to ensure that high-risk AI systems perform consistently throughout their lifecycle and meet an appropriate level of accuracy, robustness, and cybersecurity. This can include establishing accuracy metrics, communicating them to users, and conducting regular testing and audits to assess system performance. 4. Technical Robustness: Develop requirements for high-risk AI systems to be resilient against risks connected to system limitations, errors, faults, inconsistencies, unexpected situations, and malicious actions. This can involve implementing security measures, conducting vulnerability assessments, and establishing incident response plans. 5. Conformity Assessment Procedures: Define conformity assessment procedures for high-risk AI systems, including internal control checks and assessment of quality management systems and technical documentation. In cases where harmonized standards or common specifications are not available, involve notified bodies in the conformity assessment process. 6. Quality Management System: Establish a sound quality management system for AI system providers, ensuring compliance with relevant regulations, conducting conformity assessments, maintaining documentation, and implementing post-market monitoring systems. Public authorities using high-risk AI systems should adopt and implement quality management rules as part of their own systems. 7. Corrective Actions: Require providers to take necessary corrective actions if a high-risk AI system is found to be non-compliant with the requirements outlined in the regulations. This can include implementing remediation plans, conducting root cause analysis, and addressing any identified issues promptly. 8. Manufacturer Responsibility: Clarify the responsibilities of manufacturers of final products that incorporate high-risk AI systems, ensuring compliance with relevant sectorial legislation and the regulations outlined in the document. 9. Regulatory Sandboxes: Consider the use of regulatory sandboxes as a means to promote AI innovation while ensuring compliance with regulations. This can involve creating controlled environments for testing and experimentation, allowing for the identification and mitigation of potential risks. 10. Collection and Use of Expertise: Establish mechanisms for collecting and utilizing expertise from various stakeholders, including academics, businesses, social partners, non-governmental organizations, member states, and citizens. This can involve forming expert groups, conducting consultations, and leveraging external expertise to inform regulatory decision-making. It is important to note that this suggested control framework should be tailored to the specific requirements and context of the financial institution and the regulations it needs to comply with. Additionally, ongoing monitoring, review, and adaptation of the control framework should be conducted to ensure its effectiveness and alignment with evolving regulatory requirements | Certainly, to align with the regulatory requirements outlined in the EUAI document, a comprehensive control framework should be established, encompassing various aspects of AI system development, deployment, and monitoring. Here’s a recommended framework: 1. Risk Assessment and Classification Controls § Risk Identification: Implement a process for identifying and classifying AI systems based on their risk level, especially for high-risk systems. § Risk Management Plan: Develop comprehensive risk management plans for each AI system, focusing on mitigating identified risks. 2. Data Governance and Quality Controls § Data Quality Assurance: Establish procedures to ensure data accuracy, completeness, and relevance. § Bias Detection and Mitigation: Implement systems to regularly test for and mitigate biases in datasets. § Data Documentation and Traceability: Maintain detailed records of data sources, collection methods, and processing steps. 3. Compliance with Prohibited Practices § Compliance Checks: Regularly review AI practices to ensure they do not fall under prohibited categories as outlined in Article 5. § Ethical Guidelines and Training: Develop ethical guidelines and conduct regular training for staff on prohibited AI practices. 4. Transparency and Disclosure Requirements § Transparency Protocols: Establish protocols to ensure transparency in AI decision-making processes. § Information Disclosure: Set up systems for disclosing information about AI system capabilities and limitations to relevant stakeholders. 5. Governance and Oversight § Internal Governance Structure: Create an internal governance structure to oversee AI development and deployment. § External Audits and Reporting: Regularly conduct external audits and comply with reporting requirements to regulatory bodies. 6. Technical and Operational Safeguards § System Security: Implement robust security measures to protect AI systems from unauthorized access and cyber threats. § Operational Resilience: Ensure operational resilience of AI systems, including backup and recovery processes. 7. Human Oversight Mechanisms § Human-in-the-Loop Systems: Where applicable, incorporate human oversight in AI decision-making processes. § Training for Human Supervisors: Provide comprehensive training for personnel responsible for overseeing AI systems. 8. Consumer and User Protection Measures § User Rights Protection: Implement measures to protect the rights of users and consumers, as per EUAI regulations. § Feedback and Redress Mechanisms: Establish channels for user feedback and redress mechanisms for grievances related to AI systems. 9. Continuous Monitoring and Improvement § Performance Monitoring: Continuously monitor the performance and impact of AI systems. § Regular Updates and Improvements: Regularly update AI systems and practices based on monitoring insights and regulatory changes. 10. Documentation and Record Keeping § Comprehensive Documentation: Maintain thorough documentation for all aspects of AI system development and deployment. § Record Retention Policies: Implement policies for the retention and management of records in compliance with regulatory requirements. This framework is designed to ensure compliance with the regulatory requirements outlined in the EUAI document, with a focus on risk management, data governance, ethical considerations, transparency, and continuous monitoring. It should be tailored to the specific context and operations of your organization, keeping in mind the dynamic nature of AI technologies and regulatory landscapes. |
Start using RIG today
While both RIG and ChatGPT offer valuable AI capabilities, RIG stands out as the clear champion for regulatory compliance. Operating in a secure, client owned environment its sector focus and superior understanding of legal and regulatory language, combined with its document handling capabilities explains its “must-have” status amongst early tech adopters who were priorly struggling to navigate the ever-changing regulatory landscape.
At speeds 200-300 times faster than manual information processing, 24/7 availability and with a accuracy rates well over 90%, organisations are not only benefiting from unbiased outputs and insights generated, but also experience substantial cost reduction effects across departments and mitigated risks.
Contact FinregE today and experience the difference of regulatory AI built for real-world compliance.